5 Best Practices for Optimizing Your Systematic Review
Introduction
Systematic reviews are viewed as the gold standard for evidence-based research. Used in a wide variety of healthcare fields including health economics outcomes research (HEOR), regulatory compliance, guidelines development, and evidence-based medicine, they are also gaining traction as a policy development tool in social science fields such as education, crime and justice, environmental studies, and social welfare.
Regardless of why you’re conducting a systematic review, chances are there are some parts of the review process that aren’t as efficient as you’d like them to be. This guide presents five pragmatic and easy-to-use best practices for screening and data extraction to help to make these processes faster and easier while producing cleaner, better structured, and easier to analyze data.
We’ve worked with hundreds of review groups over the years, and it’s been our observation that these two processes, in particular, are often excessively time-consuming, resource intensive.
Why focus on screening and data extraction for optimizing the review process? We’ve worked with hundreds of review groups over the years, and it’s been our observation that these two processes, in particular, are often excessively time-consuming, resource intensive and more error prone when using traditional systematic review methods. Even small changes to your approach in these areas can lead to much better results with less work for your team.
So that we’re all on the same page, let’s start with a brief overview of screening and data extraction and where they fit in the systematic review process.
Screening + Data Extraction in Traditional Methods are

Time Consuming
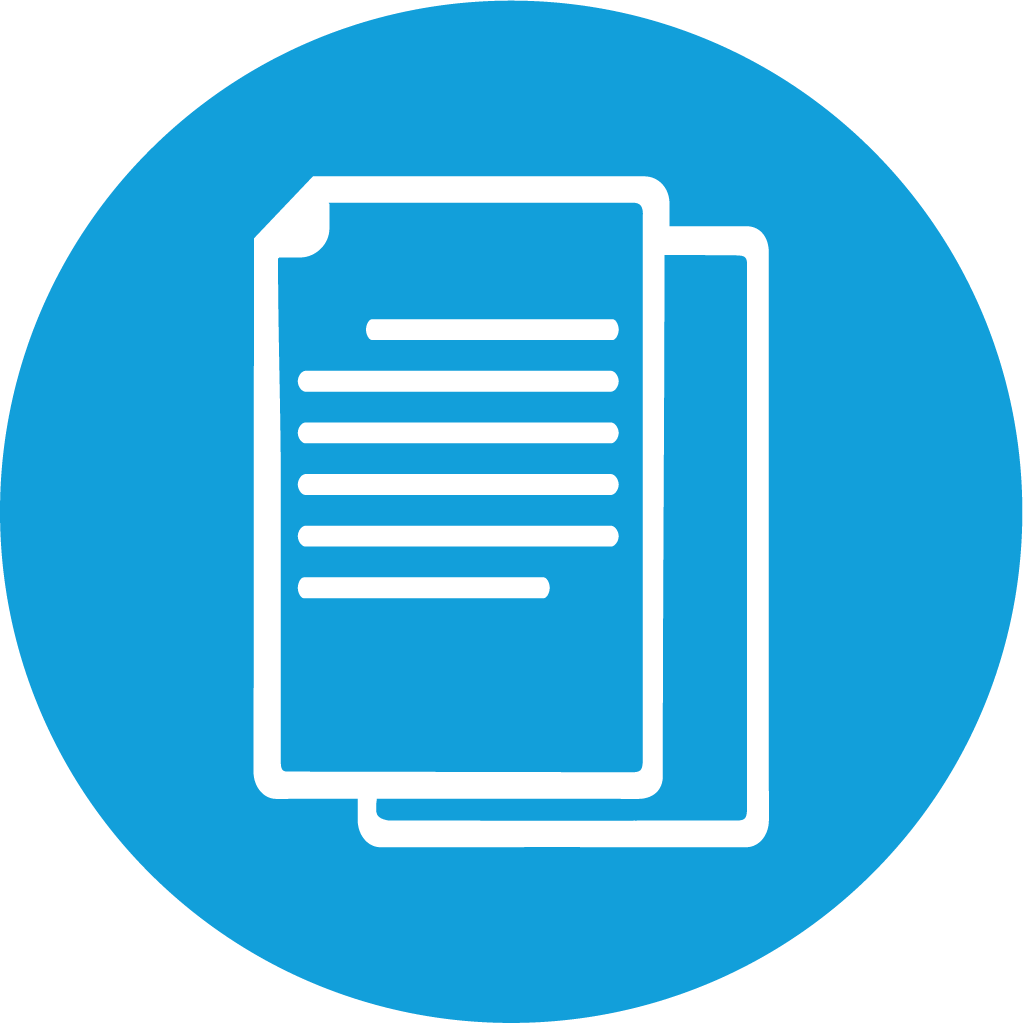
Resource Intensive
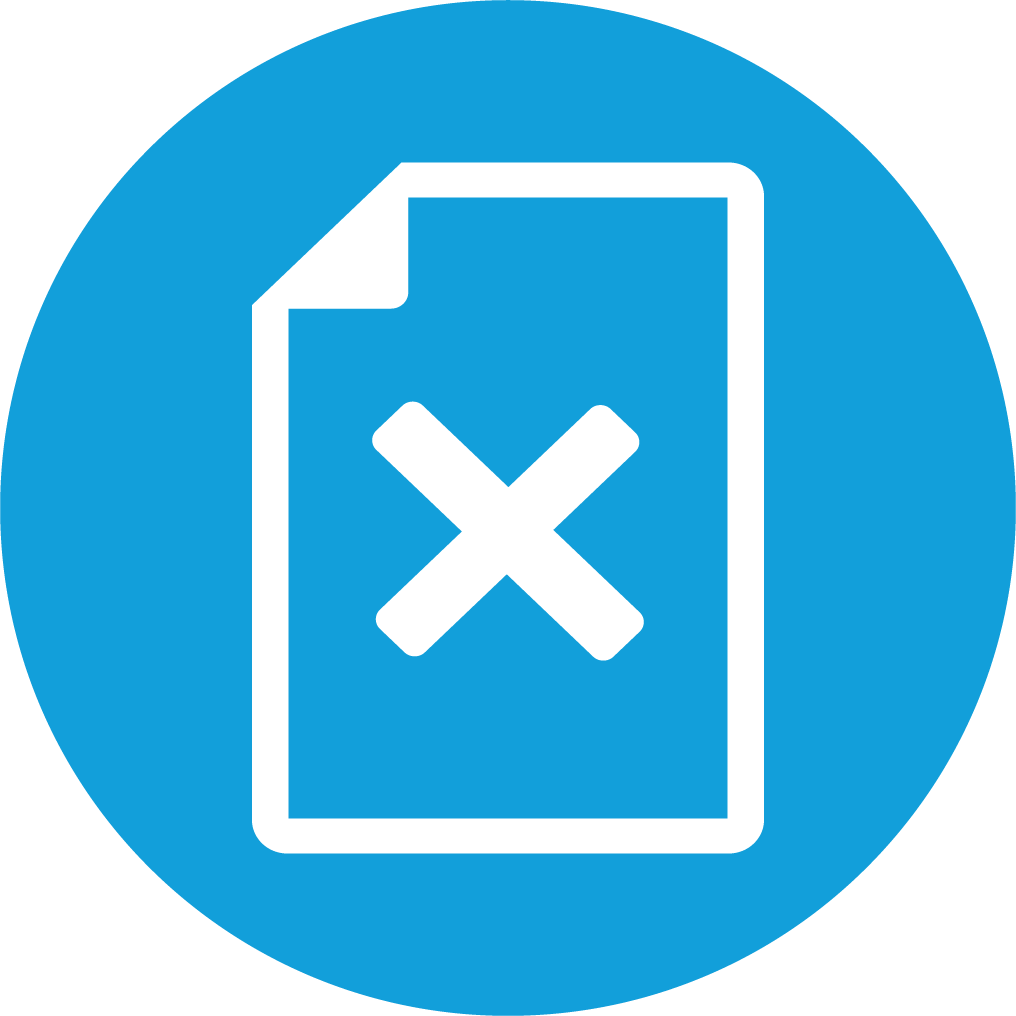
More Error Prone
Overview of the Review Process

Systematic reviews start with defining a research question.
Next, you develop a search strategy to retrieve published studies relating to that question. However, when you run your searches against the relevant databases, you typically end up with a large volume of references that may or may not be relevant to your paper or guidelines.
That brings us to screening, which is usually tackled in two stages. First, each article’s title and abstract are reviewed quickly to determine whether it may be appropriate for the study. During the initial screening stage, you will answer a small number of inclusion/exclusion questions, basing your responses only on the data available in the article citations and abstracts.
In the second screening stage, you will usually have access to full copies of references when completing the screening forms. This provides you with more data (the full references) with which to accurately answer inclusion/exclusion questions for articles that could not be excluded based on citation information in the initial screening phase of the study.
Next, it’s on to data extraction, where you need to read the full text of each article that survived the screening phase and answer a series of questionnaires to extract and codify the data you need for your research. The objective of data extraction is to convert the free form text of the included articles into tables of coded data that can be analyzed, synthesized and used for meta-analysis.
So, how do you ensure that you and your organization are conducting systematic reviews in the most optimal way possible? In our experience, the more consistent your processes are, the better your end result will be.
Even within the same organization, it’s common to find as many different review processes as there are groups conducting reviews. But what happens when a team member moves to a new project? How long does it take to get them up to speed? Without standard processes, there can be a lot of time wasted on re-learning.
Consistency is also your secret weapon in an audit situation. Following the same processes and using the same form templates from study to study will make it easier to navigate what you did and why you did it during an audit of your work.
The five tips in this guide are all designed to help you bring consistency and efficiency to the screening and data extraction phases of your review.
Use Closed-Ended Questions
Often you will see free-form text fields used to capture things like study type, publication language, or country. Generally speaking, if the set of all possible answers to these questions can be determined in advance, the practice of reviewers typing in text answers is suboptimal.
Allowing people to type in text answers tends to result in different variations of the same answer. Spelling, acronyms, abbreviations and punctuation leave a lot of room for inconsistent data entry. For example, “United States” might also be entered as U.S., US, or USA for four different ways of typing the same answer – and that’s assuming there are no typos.
Why Closed Ended Questions Are Better:
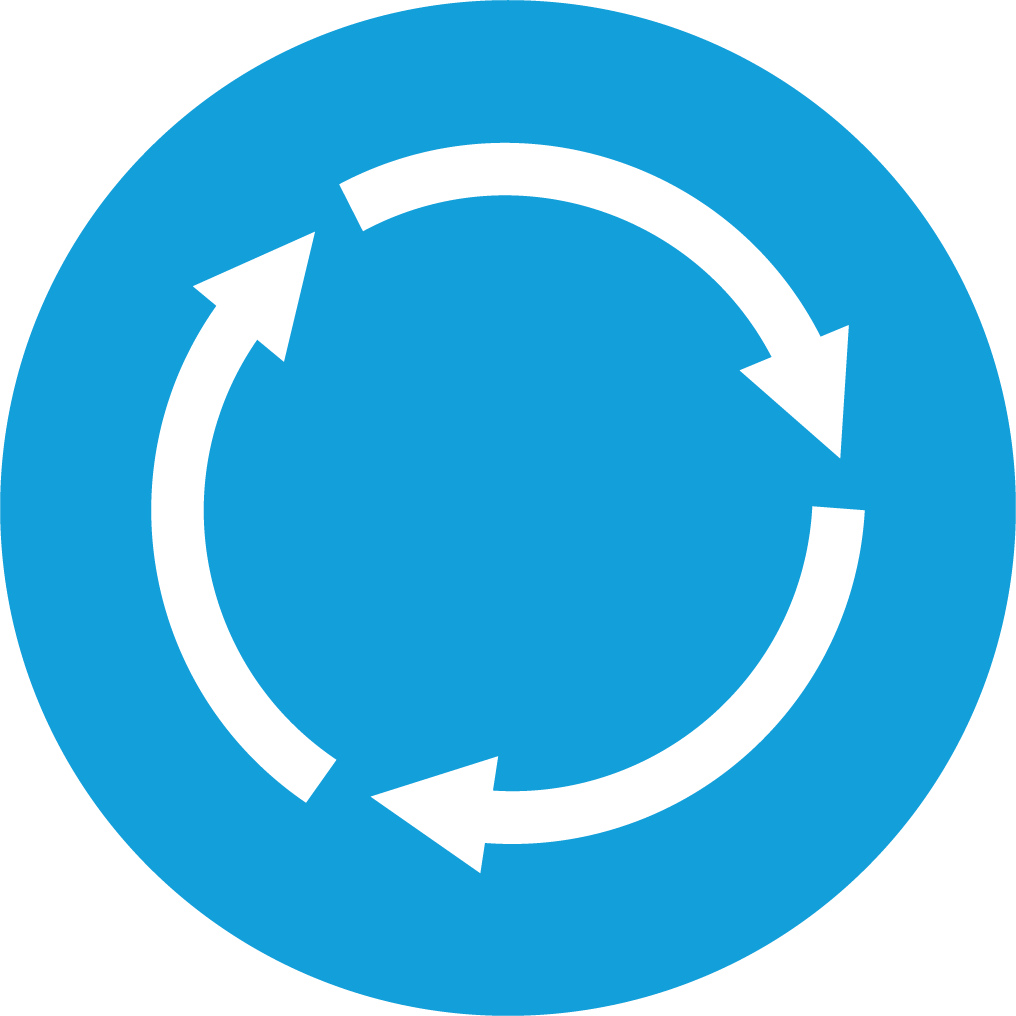
Consistency
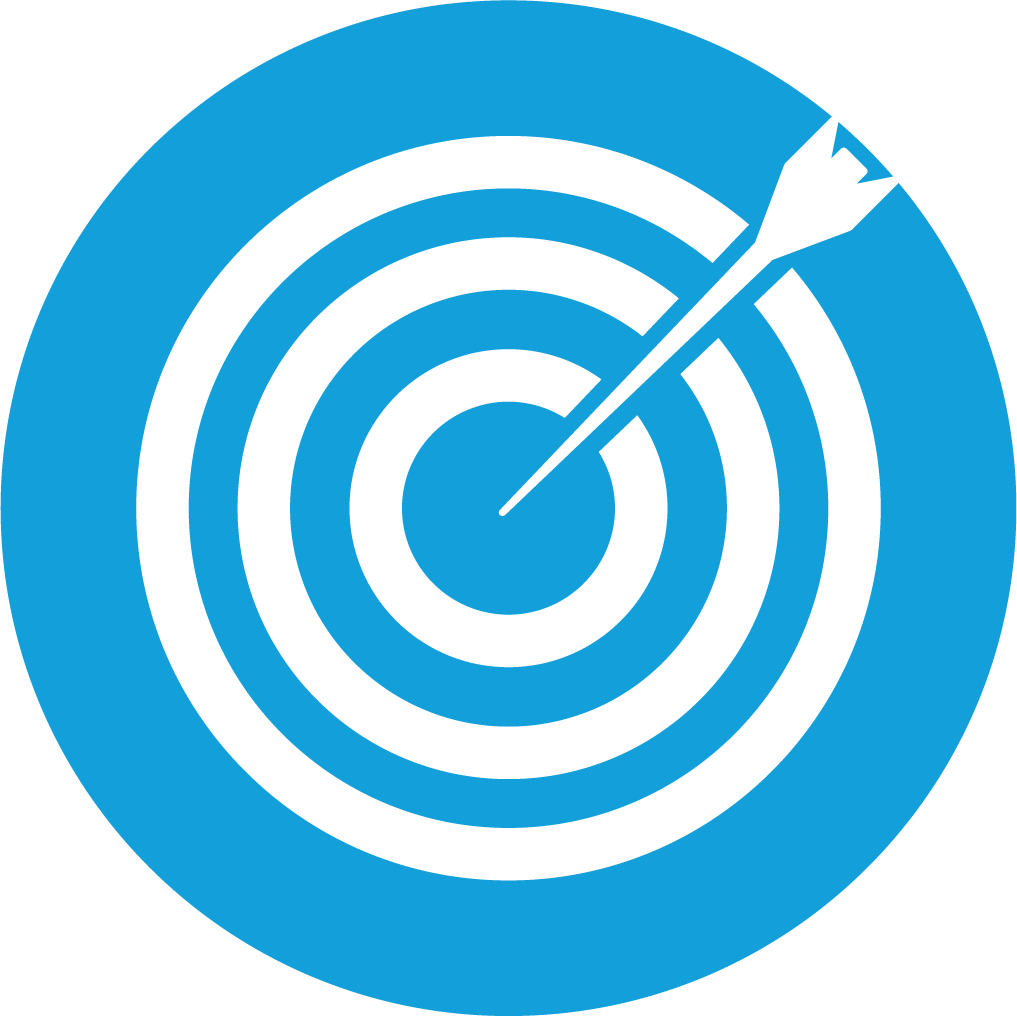
Accuracy
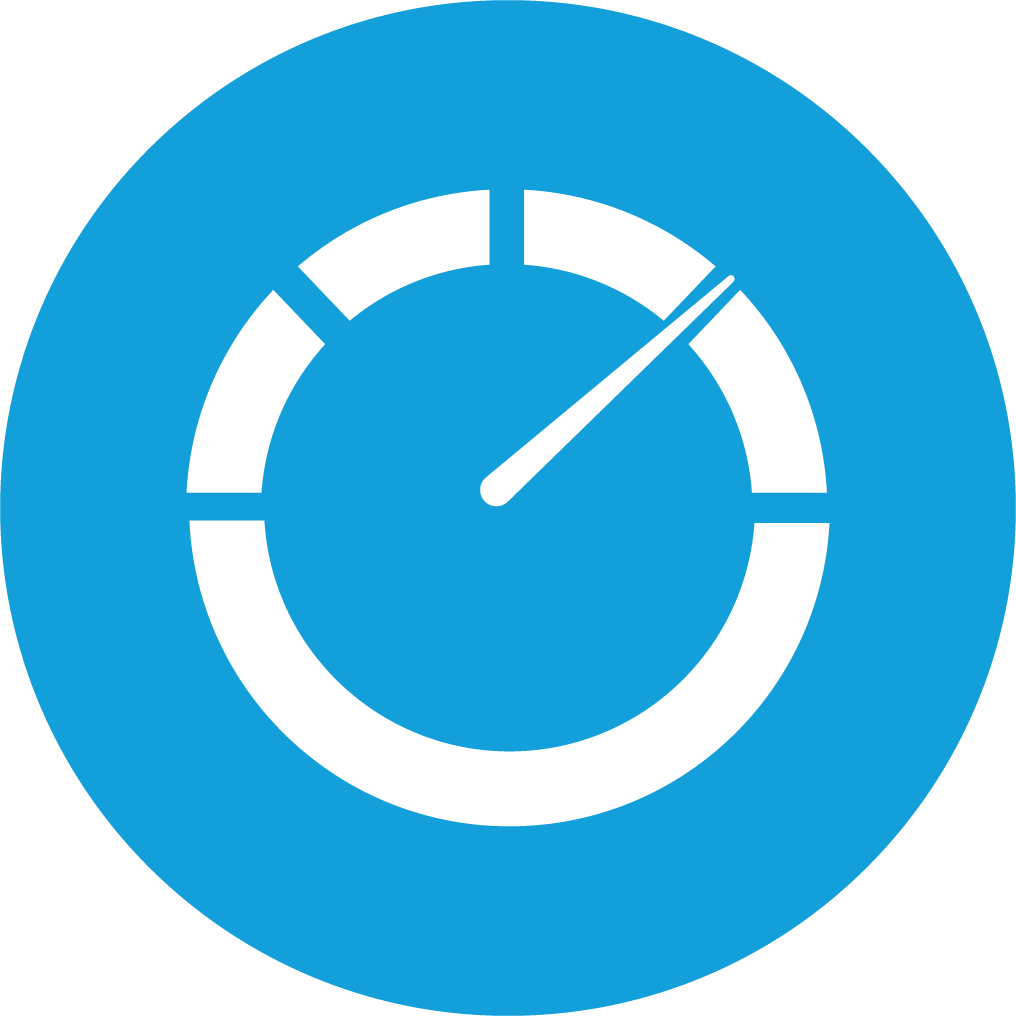
Speed
The problem with these manually typed responses is that you can’t sort, search, or compare this data effectively. Data management tools will see this as four different answers – even with just a slight difference in punctuation.
For this data to be useful for analysis, you will have to go in and do some cleanup. As you can imagine, every time your data is touched, you add time to your review and increase the risk of introducing errors. Why not just get the reviewers to enter it right the first time?
There are several question types that lend themselves particularly well to closed-ended questions, including dropdown lists, checkboxes, and multiple choice. For the “United States” example above, if you had used a multiple choice list for the Country question, the answer would have been entered the same way every time and your data set for this question would not require any cleaning.
When you’re creating a closed-ended question, it’s best to carefully consider your set of responses to try to cover all the bases. But what if there’s an answer that you haven’t anticipated and is therefore not on the list? This problem can be solved by adding an “Other” option and a text field to capture any outliers – but of course, as soon as you do that, you’re opening yourself up to inconsistencies and errors again.
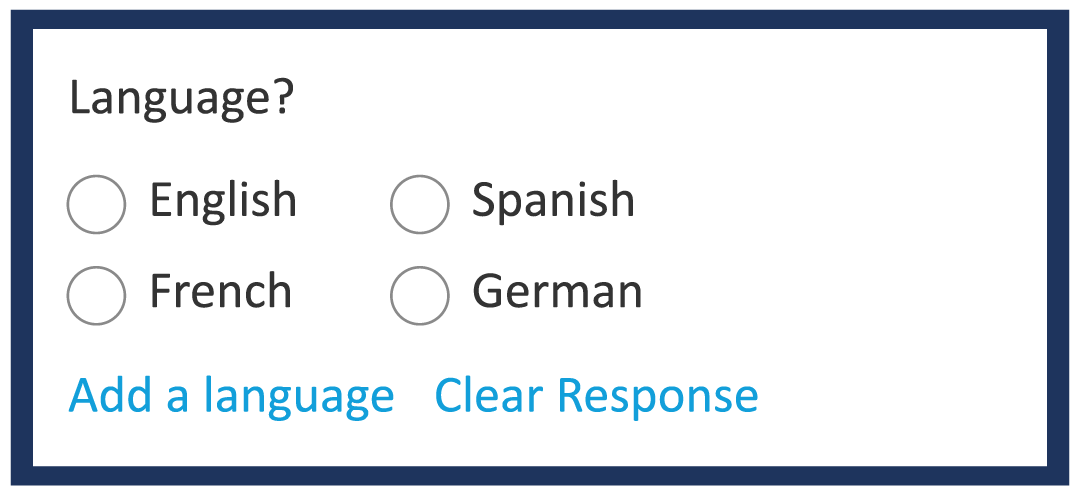
Depending on the software you’re using, your reviewers may be able to add additional closed-ended responses to form questions as they review, thus expanding the list of options as needed, for the whole team, while keeping the values consistent. Take advantage of this functionality if it’s available to you.
The bottom line is, the less you ask your reviewers, the cleaner your data will be.
Validate Data at the Point of Entry
Of course, there will always be questions that can’t be closed-ended, for which reviewers must type in answers. For these cases, there are a few things you can do to guide reviewers to give you the data you want, in the format you need.
One example that frequently comes up is numeric input for things like subject counts or age. For numeric fields, you have a few options to help ensure the captured data is clean.
First, you can use in-form validation to force the user to enter only numeric characters into the field. This is particularly useful in preventing the entry of units of measurement (e.g. cm, ml) which will mess up any mathematical operations that you want to perform on this field at analysis time. It’s much better to have a separate closed-ended question to capture units, allowing you to perform mathematical operations on the data without manual clean up.


The second thing you can do with numeric fields is use in-form validation to enforce minimum and maximum values. For example, an age of less than 0 or greater than 120 is almost certainly wrong. By setting up your question so that the value entered must be within a certain range, you can catch obviously incorrect entries while you still have the reviewer’s attention and have them correct it while it is easy to do so.

Another simple thing you can do to help focus reviewers on the data is to put limits on text boxes (so they can’t cut and paste an entire paragraph when you only have space for 50 characters!)
Finally, no discussion about validation would be complete without touching on dates, the data type that is perhaps the most inconsistently formatted. There are simply too many ways to record dates, and they vary from country to country, organization to organization, and even person to person.
To capture dates consistently:
- Use separate numeric fields for day, month, and year, or
- Use a date picker that always saves date entries in the same format (almost like a closed-ended question)
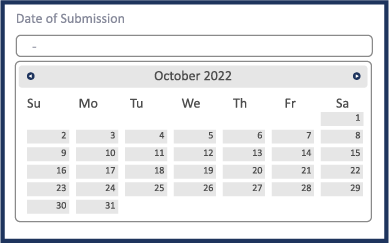
Without some enforcement of format, you are almost certain to end up with date issues, especially if you have more than a few reviewers. Using one of these techniques should help.
Manage Hierarchical Repeating Datasets Efficiently
A long-standing challenge in the systematic review community has been how to design a data extraction form, or forms, to capture related, repeating blocks of information.
Some extracted data is simple and only occurs once in a paper – the study type or setting, for example.
Other types of data, however, may be repeated throughout a paper. Studies may examine multiple outcomes, and each outcome may have multiple study arms, with results for each study arm measured across multiple time periods. The number of each of these data subsets can vary widely from study to study.
The simplest and most common approach has traditionally been to extract the data directly into a spreadsheet, inserting new rows and columns as relevant data is found in the papers.
However, as you can imagine – and may have experienced yourself – with a column for each possible combination of repeating data, your spreadsheet can quickly become monolithic and impossible to analyze. We call this a data management nightmare!
The solution? For each recurring data type, create a separate form or table. Reviewers can complete as many forms or tables as they need for a given study, instead of trying to anticipate widely from study to study.
When using this approach, remember to link the child dataset with its parent (e.g. by having a question in the child form that names its parent) to retain the context of the information. As long as the hierarchy is maintained, you’ll end up with a database-style table that will be dramatically easier to manipulate and analyze.
Bad

Good

Don’t Ask for More Than You Need
Clutter has a way of creeping into our lives, and systematic reviews are far from immune. One way to reduce clutter in your final data set is to design your data collection forms after the tables are mapped out for your final output.
It may seem counterintuitive, but crafting your forms last will ensure you know exactly what information you need so you can put the right questions, with the right wording and the right format, on your forms.
Your systematic review software may allow you to include dynamic forms with conditional hide/show logic, which can be extremely useful for ensuring you get all the required data without any unnecessary information. Based on previous answers, dynamic forms show you only the questions that are applicable – you can even hide a whole form if it doesn’t apply.

Standardize for Reuse
Chances are, if you’re working on multiple reviews, your study instruments (i.e. forms) are the same or similar for each one.
If not, here’s why you should consider standardizing them.
Standard forms – even if they require minor tweaks from project to project – can reduce the errors and increase the speed of your review team. Because you’re not reading a brand new form every time, you will quickly become much more efficient when filling them out.
Stop!
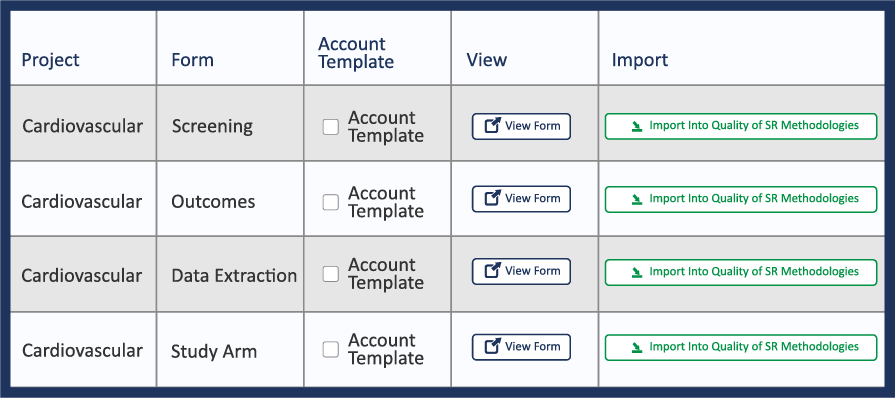
Tag Excluded References So You Can Find Them Later
So far, we’ve shared five tips to help improve the speed and quality of your screening and data extraction. But what happens to the references that didn’t make the cut?
If you’re like most review groups, excluded references are quickly forgotten once it has been determined that they aren’t a good fit for your project. But during the screening process, it’s common to come across references that aren’t relevant to your current review project, but could be useful in another review or are of general interest to the screener.
Rather than just tossing these references into the “excluded” pile, try adding a question or two to your screening form to allow reviewers to tag them for future use.
Here are three examples of tags you can use on excluded references to help you find them later:
- Keep as background material”
- “Potentially Useful for Study X”
- “I’d like to Read This”
1. “Keep as background material”
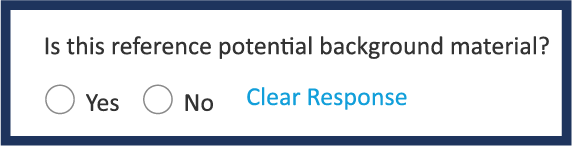
Even if a reference doesn’t meet your inclusion criteria, it may still contain relevant background material for your review.
Let’s say you find a paper that contains substantial referenceable material, but was published outside of the target date range for the study. You can’t use the results in your findings because it does not meet the exact inclusion criteria for your review.
This is the perfect opportunity to use a tag to identify this paper as background material. In doing so, you can easily refer to it when preparing the study write-up even though it won’t appear in your tables or meta-analysis.
2. “Potentially Useful for Study X”
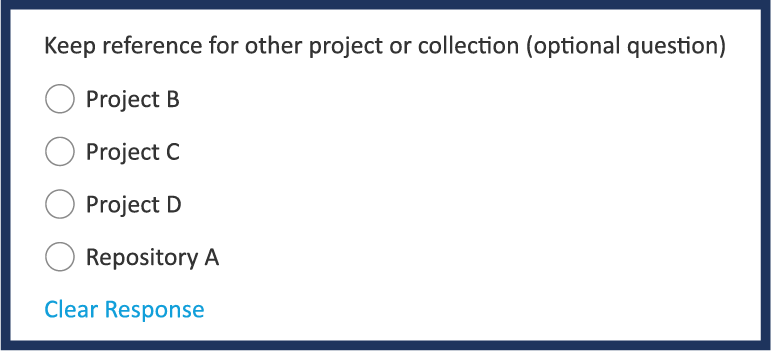
Many groups run multiple systematic review on closely related subjects. As such, it is not uncommon for searches to turn up references that don’t meet the inclusion criteria for the current review but are likely candidates for one of the other reviews being conducted. An informed screener can tag these articles for easy retrieval from the excluded pile for use in other systematic reviews that the group may be working on.
3. “I’d Like to Read This”

Don’t interrupt your work flow to read interesting material if it’s not related to your project – tag it for your “to read” list!
As reviewers make their way through hundreds or thousands of titles and abstracts, it’s highly likely they’ll come across material that looks interesting but is unrelated to the review they are working on.
By tagging this material, you can quickly save it for later and avoid distraction during the screening process.
Give it a try – you might just end up with a head start on your next review project.
Conclusion
Implementing these five tips won’t make your systematic reviews completely painless, but they should improve your screening and data extraction processes.
5 Best Practices
- Use Closed-Ended Questions
- Validate Data at the Point of Entry
- Manage hierarchical repeating datasets efficiently
- Don’t Ask For More Than You Need
- Standardize for Re-use
Bonus: Tag excluded references so you can find them later
All of these tips have one thing in common: they will help you to improve the efficiency and consistency of your review process.
Completing a review faster, with fewer errors… who doesn’t want that?